Alternative payment models (APMs) have become an increasingly common form of reimbursement in the U.S. healthcare system. The Centers for Medicare and Medicaid Services (CMS) has been clear about its goal to see more providers participating in APMs,1 and the Medicare Access and CHIP Reauthorization Act of 2015 (MACRA) introduced explicit rewards for providers who meet certain APM participation thresholds. Traditional Medicare has heavily promoted its own APMs, such as the Medicare Shared Savings Program (MSSP). Commercial APMs are also quite prevalent—in fact, Health Affairs estimated that, in 2018, commercial accountable care organization (ACO) contracts accounted for more than half of all ACO-covered lives.2
Use of APMs in the Medicaid market has been lower. Despite accounting for approximately 22% of the U.S. population,3 Medicaid participants in ACOs account for only 10% of ACO-covered lives in 2018, according to Health Affairs. In this paper, we focus on some of the challenges that Medicaid payers (including states and managed care organizations) face when trying to establish APMs with providers. In particular, we focus on shared savings/risk contracts based on total cost of care (see the Overview of TCOC Models sidebar), as opposed to bundled payments or episode models.
There are a variety of reasons why these models can be more difficult to implement and operationalize in Medicaid, compared to the commercial or Medicare environments. Understanding these nuances and building strategies to address them will be critical to the success of Medicaid APMs.
Overview of TCOC Models
In this paper, we focus on the total cost of care (TCOC) model, which includes (with few exclusions) all services covered by the payer. At a high level, a TCOC model holds the provider (or ACO) accountable to the total cost (and quality) outcomes for an attributed population. How this financial accountability is calculated varies at a granular level but tends to follow a general structure:
- A set of members (attributed population) is assigned to the provider or ACO
- For each provider or ACO, a TCOC expenditure target is established for the performance year
- Often using historical claims experience (baseline), trended and adjusted forward to the performance year
- Alternatively, this could be set as a percentage of premium or capitation rate
- Actual expenditures are compared to the target, resulting in a cost savings or loss
- The provider or ACO shares in the savings or loss, often adjusted based on quality performance
1. Attribution
The goal of attribution is to assign responsibility for outcomes and the cost of care for a patient population to a provider or group of providers (like an ACO). In many total cost of care models, patients are attributed to a provider based on use of primary care services, for example having the most evaluation and management (E&M) visits during a given time period. Because Medicaid beneficiaries are more likely to access primary care through the emergency room (ER), urgent care, or perhaps not at all, it can be difficult to appropriately attribute a patient to a provider who is realistically able to manage the patient’s care.
Figure 1 summarizes the percentage of non-dual individuals—including Temporary Assistance for Needy Families (TANF), Supplemental Security Income (SSI), and expansion populations—who had at least one E&M visit during a calendar year and the percentage of individuals who had at least one ER visit but no E&M visits. This is based on analysis of the 2016 Medicaid, commercial, and Medicare fee-for-service (FFS) markets in the same set of three states.4 The Medicaid market had the lowest percentage of patients with E&M visits and the highest percentage of patients with ER visits but no E&M visits. This latter set of patients likely represents an opportunity to manage care and reduce costs, but it is difficult to determine which physicians should be at financial risk for them under a total cost of care arrangement.
Figure 1: Percentage of Patients With at Least One E&M Visit and/or ER Visit During a Calendar Year
Metric | Market | ||
Commercial | Medicare FFS | Non-dual Medicaid | |
Percentage with E&M visit | 72% | 87% | 69% |
Percentage with ER visit and no E&M visit | 1% | 2% | 6% |
Note: Values have been rounded. Based on analysis of 2016 markets in the same set of three states. See appendix for data sources and methodology. |
THE TAKEAWAY: Understanding how different approaches to attribution may impact outcomes for a Medicaid APM provider will be an important factor in ensuring that financial incentives are aligned. Including member choice in the attribution process can help ensure patients are aligned with the provider who is most responsible for their care. Attributing members without any E&M services on the basis of geography or some other method can expand the population covered by the APM, but this could result in providers having financial risk for patients with whom they do not have established relationships.
2. Claims volatility
One challenge with any total cost of care model is that providers inherently take on some level of insurance risk due to random claims fluctuation that can influence results. This is true in the Medicare ACO models, which is why CMS uses a minimum savings rate (MSR) that varies by population size to limit its payments for “false positives.” This is likely to be more pronounced in Medicaid because of challenges with attribution (discussed above), beneficiaries moving in and out of Medicaid, and a higher prevalence of zero-dollar claimants.
We illustrate this dynamic by examining the variation in year-over-year medical (non-Rx) costs per member per month (PMPM) for simulated 10,000-life populations in the Medicare, non-dual Medicaid, and commercial markets.5 We found that the standard deviation of the risk-adjusted PMPM cost trend was 2.5% in Medicare, 4.1% in Medicaid, and 4.5% in commercial. Figure 2 presents the distribution of simulated trends, relative to the overall average. For the Medicare population, the risk-adjusted trend was within +/-5% of the average in approximately 95% of the trials; for Medicaid, this occurred in only approximately 80% of the trials. In other words, for a Medicare ACO with 10,000 lives, the actual claims costs will be within 5% of the expected claims costs about 95% of the time, but for a Medicaid ACO of the same size, the actual claims costs will only be within 5% of the expected claims costs about 80% of the time.
Figure 2: Range of One-Year Risk-Adjusted PMPM Trends, Relative to Market Average: Simulated 10,000-Life Groups
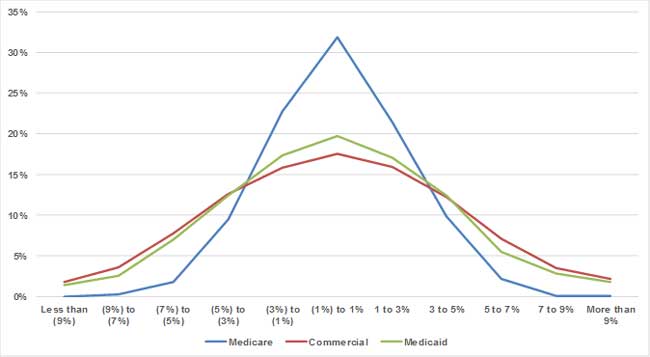
Note: Based on analysis of 2015-2017 markets in the same set of three states. See appendix for data sources and methodology.
For the Medicaid population, we did observe lower volatility among disabled cohorts compared to TANF and expansion cohorts. Volatility among the disabled cohort was similar to the Medicare population, and volatility among the TANF and expansion cohorts was slightly higher than the commercial population.
One final note: this analysis does not account for additional volatility that is introduced when populations are attributed to individual providers. Attributed populations have even higher turnover rates, and this is exacerbated in Medicaid because of the challenges with accurately attributing beneficiaries to providers.
THE TAKEAWAY: Use of established actuarial mechanisms for addressing random claims variation, such as stop-loss thresholds and carve-outs of high-cost services, is an important component of a Medicaid APM. Consultants with expertise in this area can use state-specific data to model the sensitivity in financial outcomes for a given population size and stop-loss threshold. This information can be vital in developing meaningful parameters in a Medicaid APM.
3. Risk adjustment
Most total cost of care models start with a baseline population (and claims) and then trend and adjust this forward to the performance year to establish the target (or budget) per capita costs. Adjustment typically includes risk adjustment, which helps to account for changes in the relatively healthy acuity of the attributed population from the base year to the performance year.
A risk adjustment model produces a risk adjustment factor for each patient, based on the diagnosis code history, age, gender, and other information, to predict that person's cost of care (relative to an average patient) in the current year (concurrent risk adjuster) or the next year (prospective risk adjuster). Risk adjusters are commonly used by CMS and state Medicaid agencies for adjusting healthcare premiums or capitation rates such that payers with chronically sicker populations would get higher rates than payers with healthier populations. Risk adjusters are also commonly used to make similar adjustments in APMs when establishing target budgets.
A risk adjustment model is calibrated to a specific population type (Medicare, commercial, Medicaid) with coefficients varying by age, gender, disease categories, eligibility status, and other factors. There are many different risk adjustment models in the marketplace, including publicly available models designed for Medicare (CMS-Hierarchical Condition Category [HCC] risk adjuster) or Patient Protection and Affordable Care Act (ACA) exchange (U.S. Department of Health and Human Services [HHS]-HCC risk adjuster) populations as well as commercially available models such as Milliman Advanced Risk Adjusters™ (MARA™). The most common risk adjustment model in Medicaid managed care programs is the Chronic Illness and Disability Payment System (CDPS),6 along with the complementary MedicaidRx model that uses prescription drug history. This model is similar to the CMS-HCC model but focuses on chronic conditions that are more prevalent in disabled Medicaid populations.7
There are some key considerations when applying a risk adjustment model for a Medicaid APM. By default, the CDPS model includes different sets of coefficients for disabled and nondisabled populations. One approach is to develop risk-adjusted targets separately for the disabled and nondisabled populations (or possibly a more granular level). Another approach would be to normalize the risk scores such that the disabled and nondisabled populations are both on the same 1.00 risk score basis. Alternatively, actuaries or other subject matter experts can apply machine learning algorithms to responsibly recalibrate the coefficients to reflect the characteristics of the population included in the APM.
THE TAKEAWAY: Disease and demographic coefficients should be reviewed for appropriateness relative to the populations and services covered by the APM. Regardless of the weights or model used, encounter data should be reviewed thoroughly to ensure consistent coding practices across managed care organizations (MCOs) and over time so that changes in morbidity can be measured accurately.
4. Alignment with managed care
Although some Medicaid APMs are negotiated between MCOs and providers, many are run by state Medicaid agencies. For instance, in Ohio, physician practices can choose to participate in the Comprehensive Primary Care (CPC) program, which is operated by the Ohio Department of Medicaid.8 Practices are attributed a panel of patients across all MCOs, plus fee-for-service members. The total cost of care is measured across the entire attributed panel.
In these instances, it can be difficult to cleanly align the program with the existing managed care environment.
- The MCOs are already incentivized to lower the total cost of care for their members and generally have utilization management and other programs in place to achieve this goal. This creates a risk that providers could be rewarded for savings that were actually driven by the MCO activities. In some cases, MCOs are responsible for making shared savings payments to providers, and therefore MCOs will want to be assured they are not paying the provider for savings the MCOs created. However, MCOs could indeed benefit from claims cost savings generated by providers without having the administrative burden of setting up the APM.
- MCOs often sub-capitate certain services, which means that MCOs do not benefit financially from lower utilization of these services (at least in the short term). However, if repriced encounter claims for these services are included in the total cost of care calculations rather than the actual sub-capitation payments, the MCOs may end up paying shared savings without reaping any benefits from lower expenses.
- States face a similar challenge in that any reductions in total cost of care for managed care members will not accrue to the state immediately, because managed care capitation rates are established prospectively. For instance, if the base experience period for capitation rates is two years prior to the payment year, savings generated in 2018 for managed care members will not be reflected in capitation rates until 2020.
THE TAKEAWAY: If incentives are aligned, providers engaged in an APM have the potential to reduce the cost of care for Medicaid beneficiaries beyond what MCOs can achieve alone. In order to get buy-in from all stakeholders and minimize unintended consequences, states should carefully consider interactions with the managed care environment when implementing or making changes to a Medicaid APM and when developing managed care capitation rates.
5. Quality metrics
APMs almost always include some link to quality of care to ensure that costs are not prioritized over outcomes and patient experience. Quality can be difficult to measure, however. This is true even when data is relatively clean and standardized, like in the Medicare fee-for-service world. Medicaid encounter data may be less complete and accurate for a number of reasons (e.g., needing to pass through both a MCO's and a state’s claims adjudication systems), which poses additional challenges for evaluating quality metrics for an APM. However, it should be noted that CMS introduced stricter requirements on encounter data quality in the 2016 Medicaid Final Rule, including penalties that went into effect for contracts starting July 1, 2018, or later.9
Even assuming perfect data quality, social determinants can make it harder for providers to influence quality outcomes for Medicaid patients. For example, patients may have difficulty finding convenient transportation for routine physician visits or to fill maintenance medications, and those with unstable housing situations may be less likely to follow an optimal post-discharge recovery plan after an inpatient stay.
THE TAKEAWAY: When establishing a set of quality measures for use in a Medicaid APM, it is important to understand these confounding factors to ensure alignment within the APM. Using a phased-in approach (increasing the impact of quality on financial results over time) or taking a tiered approach (paying for performance for some measures and paying for reporting on others) can help facilitate the development of a quality measures program that works best for all stakeholders with less risk of frustrating providers who may feel they have little control over outcomes.
6. Service carve-outs
In the long run, higher use of certain types of services, such as annual physicals, immunizations, and dental cleanings, may lead to better health and lower costs.10,11 As a general rule, APMs should avoid incentivizing providers to reduce utilization of these types of services. This is particularly critical in Medicaid, where members are often high utilizers of emergency services and low utilizers of primary care and other preventive services. Additionally, Medicaid includes some services not covered by other payers, such as high-intensity behavioral health or nonemergency transportation. Medicaid APMs may therefore want to carve out certain services from the total cost of care calculations.
Long-term services and supports (LTSS) is a major driver of costs for Medicaid programs. The use of managed care for LTSS is growing rapidly; in August 2017, 24 states had some enrollment in LTSS programs (up from 16 states in 2012).12 But many states have yet to address this unique and costly population within managed care. Similarly, there are many challenges with including LTSS in a Medicaid APM—particularly because of how this population uses and accesses care—and discussing these challenges in detail is outside the scope of this paper. However, we wanted to acknowledge this is an important component of Medicaid spending and should continue to be considered in the context of APMs as each state evolves along the managed care spectrum for LTSS populations.
THE TAKEAWAY:Using data to model the potential impact to financial results of carving out certain services for a Medicaid APM is an important exercise and can be used to inform policy decisions and contracting negotiations with providers and ACOs.
7. Variation in benefits and coordination with other payers
In some Medicaid programs, different sets of services are offered to individuals depending on their reasons for Medicaid eligibility, or the services are reimbursed in different ways. For instance, certain services may be covered under an 1115 waiver for only a subset of the population. These covered services could also change over time, which is particularly problematic if the targets are set based on experience in a historical baseline period.
Additionally, many beneficiaries have dual eligibility with Medicare, meaning that Medicaid is paying only a portion of the total costs for these beneficiaries for Medicare-covered services. The coordination of benefits adds an additional layer of complexity when attempting to measure the savings generated by a provider. A simple solution is to remove these beneficiaries from all calculations, but this will reduce the total impact that the APM can have on costs and quality outcomes for the system.
THE TAKEAWAY: Excluding certain populations for a Medicaid APM is an option for handling variation in benefits and coordination with other payers. But the advantages of this approach should be weighed against potential added complexity or reductions in members covered by the APM.
Conclusion
APMs are an important mechanism for continuing to improve the value of care in the Medicaid market. However, the Medicaid population presents some unique challenges, discussed in this paper, which must be considered in order to maximize the potential outcomes of a Medicaid APM. We recommend partnering with actuarial and financial experts to help facilitate understanding of these challenges, assist with developing contracting strategies, and design APM models that have carefully considered these nuances.
Acknowledgments
The authors would like to thank Lucas Everheart, Steven Buehler, and Kevin Baumgart for their help in performing the analyses discussed in this paper.
Limitations and qualifications
The information in this paper is intended to describe challenges with Medicaid alternative payment models. It may not be appropriate, and should not be used, for other purposes. Commentary in this paper should not be considered recommendations for any specific state Medicaid agency, provider, or managed care organization.
In performing the analysis for this paper, we relied on data made available by CMS, the Milliman Consolidated Health Cost Guidelines™, and various state Medicaid agencies. We have not audited or verified this data and other information. If the underlying data or information is inaccurate or incomplete, the results of our analysis may likewise be inaccurate or incomplete.
We performed a limited review of the data used directly in our analysis for reasonableness and consistency and have not found material defects in the data. If there are material defects in the data, it is possible that they would be uncovered by a detailed, systematic review and comparison of the data to search for data values that are questionable or for relationships that are materially inconsistent.
Guidelines issued by the American Academy of Actuaries require actuaries to include their professional qualifications in all actuarial communications.
Anders Larson is a member of the American Academy of Actuaries and meets the qualification standards for performing the analyses presented in this report.
Anders Larson, FSA, MAAA, is a consulting actuary with the Indianapolis office of Milliman. Contact him at anders.larson@milliman.com.
Rebecca Johnson is a senior healthcare management consultant with the New York office of Milliman. Contact her at rebecca.johnson@milliman.com.
Zach Hunt is an actuarial student with the Indianapolis office of Milliman. Contact him at zach.hunt@milliman.com.
Appendix: Data Sources and Methodology for Simulating Claims Volatility
Analyses in this paper related to E&M visit utilization, emergency room (ER) utilization, and claims volatility were based on data from three states. The following sources were used:
- Medicare: Medicare 5% Sample Limited Data Set (LDS).
- Medicaid: Encounter data warehouse from three states, limited to non-dual population (including TANF, SSI, and expansion populations).
- Commercial: Milliman Consolidated Health Cost Guidelines Sources Database (CHSD).
For the analysis of E&M and ER visits, we identified outpatient E&M visits using Current Procedural Terminology (CPT) codes 99201 to 99215, 99381 to 99397, G0438, G0439, and G0468. Although other CPT codes are often used for attribution, we used this narrow set of commonly used codes, including some preventive services, for consistency across markets. ER visits were identified using revenue codes 0450 to 0459 and included inpatient stays that originated in the ER.
For the claims volatility analysis, we randomly selected 10,000 individuals in each market and tracked their risk-adjusted costs in two subsequent years. We used paid costs for Medicare and Medicaid markets, because those are most commonly used for total cost of care models, but we used allowed costs for the commercial market to mitigate the impact of plan design changes. Each individual’s costs were capped at $200,000 in a given year. Individuals who lost coverage in the second year were replaced by individuals from the same state and with a similar demographic profile (age, gender, rate cell, Medicare entitlement category, etc.). For the commercial population, we also required that exiting individuals were replaced by an individual of the same employer group to avoid large changes in the mix of plan designs. The risk scores were calculated on a prospective basis for all groups. The risk score models used for each population were:
- Medicare: CMS-HCC.
- Medicaid: CDPS + Rx.
- Commercial: Milliman Advanced Risk Adjusters (MARA).
1 Alternative Payment Model Framework and Progress Tracking (APM FPT) Work Group (January 12, 2016). White Paper: Alternative Payment Model (APM) Framework. Health Care Payment Learning and Action Network. Retrieved January 15, 2019, from https://hcp-lan.org/workproducts/apm-whitepaper.pdf.
2Muhlestein, D. et al. (August 14, 2018). Recent progress in the value journey: Growth of ACOs and value-based payment models in 2018. Health Affairs. Retrieved January 15, 2019, from https://www.healthaffairs.org/do/10.1377/hblog20180810.481968/full/.
3Medicaid (at https://www.medicaid.gov/medicaid/program-information/medicaid-and-chip-enrollment-data/report-highlights/index.html) estimated 73 million individuals on Medicaid, and World Population Review (at http://worldpopulationreview.com/countries/united-states-population/) estimated a total U.S. population of 327 million in February 2018
4See appendix for data sources and methodology.
5See appendix for data sources and methodology.
6The CDPS model is prepared by the University of California, San Diego. More information is available at http://cdps.ucsd.edu/.
7Gilmer, T. Risk Adjustment in Medicaid Using CDPS. ResDAC. Retrieved January 15, 2019, from https://www.resdac.org/sites/resdac.umn.edu/files/Risk%20Adjustment%20in%20Medicaid%20Using%20CDPS%20%28Slides%29.pdf.
8More information about Ohio's CPC program is available at https://medicaid.ohio.gov/provider/PaymentInnovation/CPC.
9Cunningham, J., Tressel Lewis, M., & Houchens, P. (May 2016) Encounter data standards: Implications for state Medicaid agencies and managed care entities from final Medicaid managed care rule. Milliman White Paper. Retrieved January 27, 2019, from https://www.milliman.com/insight/Encounter-data-standards-Implications-for-state-Medicaid-agencies-and-managed-care-entities-from-final-Medicaid-managed-care-rule/.
10CDC (September 15, 2017). Preventive Health Care. Retrieved January 15, 2019, from https://www.cdc.gov/healthcommunication/toolstemplates/entertainmented/tips/
PreventiveHealth.html.
11Bensley, L., VanEenwyk, J., & Ossiander, E.M. (May 2011). Associations of self-reported periodontal disease with metabolic syndrome and number of self-reported chronic conditions. Preventing Chronic Disease. Retrieved January 15, 2019, from https://www.cdc.gov/pcd/issues/2011/may/10_0087.htm.
12Lewis, E. et al. (January 29, 2018). The Growth of Managed Long-Term Services and Supports Programs: 2017 Update. Truven Health Analytics. Retrieved January 15, 2019, from https://www.medicaid.gov/medicaid/managed-care/downloads/ltss/mltssp-inventory-update-2017.pdf